Market Mix Modelling and Cost Optimization
To view our performance at glance click here
Project Overview
In a highly competitive pharmaceutical market, our client sought to optimize their marketing spend to achieve maximum revenue growth. We undertook a market mix modeling and cost optimization project to develop a precise cost equation that would guide their campaign investments for optimal returns. Leveraging advanced data science techniques, we provided actionable insights that significantly enhanced their marketing efficiency and effectiveness.

Objective
Challenges :
-
Data Complexity: Integrating and processing large volumes of data from various sources posed significant challenges.
-
Identifying Key Factors: Determining the most influential factors impacting revenue from a vast array of variables required sophisticated analysis.
-
Model Accuracy: Ensuring the predictive accuracy of the regression models to provide reliable insights and recommendations.
Goal :
-
The aim was to identify the most effective allocation of marketing spend across various channels to achieve the highest possible return on investment (ROI).
-
​To deliver a comprehensive and optimized cost equation that enables the client to strategically allocate their marketing budget for maximum revenue growth while minimizing unnecessary expenditures.
Approach
-
Data Collection: We gathered extensive data from various sources, including past marketing campaigns, sales data, and external market factors.
-
Data Preprocessing: The collected data was cleaned, normalized, and prepared for analysis to ensure accuracy and reliability.
-
Feature Engineering: Key features were identified and engineered to capture the influential factors impacting sales and revenue.
-
Model Development: We employed regression techniques using Random Decision Forest (RDF) and XGBoost to identify the coefficients of the cost equation. These models were chosen for their robustness and ability to handle complex interactions between variables.
-
Optimization: The derived cost equation was used to simulate different marketing spend scenarios, optimizing for the highest revenue with the least investment.
-
Validation: The model’s predictions were validated against actual historical data to ensure reliability and accuracy.
Results​
-
Optimized Cost Equation: We successfully developed an optimized cost equation that provided clear guidelines on how to allocate marketing spend across various channels.
-
Revenue Growth: The optimized marketing strategy led to a significant increase in revenue with reduced marketing costs.
-
Model Accuracy: The regression models, validated with historical data, demonstrated high accuracy in predicting the impact of marketing spend on revenue.
Impact
-
Increased ROI: The optimized cost equation enabled the client to achieve a higher return on investment for their marketing campaigns.
-
Cost Savings: By minimizing unnecessary marketing spend, the client saved substantial costs, which could be reallocated to other strategic initiatives.
-
Strategic Insights: The project provided valuable insights into the most effective marketing channels and strategies, informing future marketing decisions.
​
Overall Accuracy

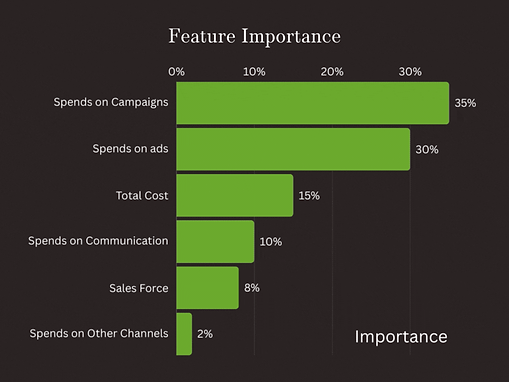
